UAS for Precision Agriculture: State of the Art, Challenges, and Future Perspectives
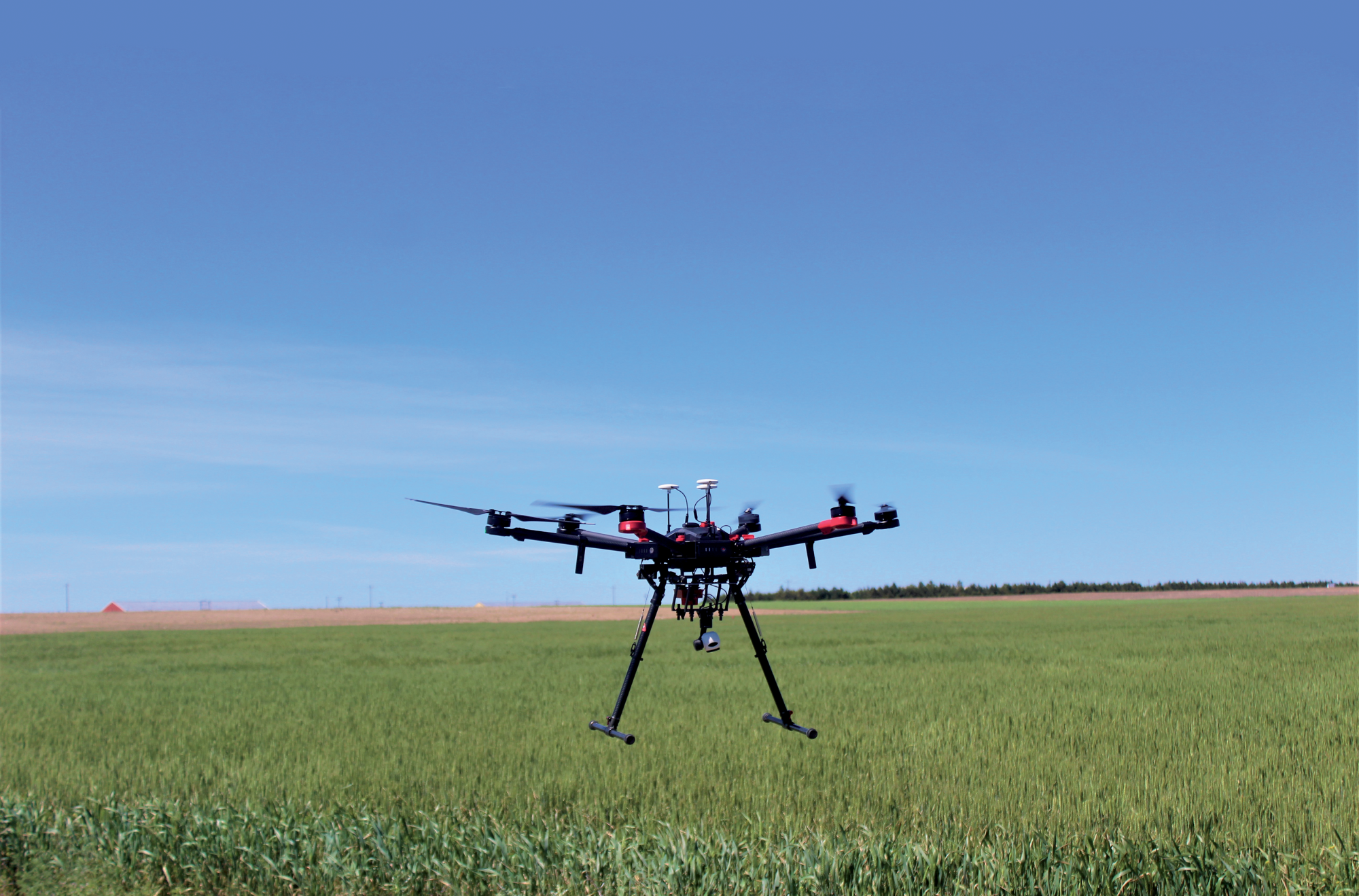
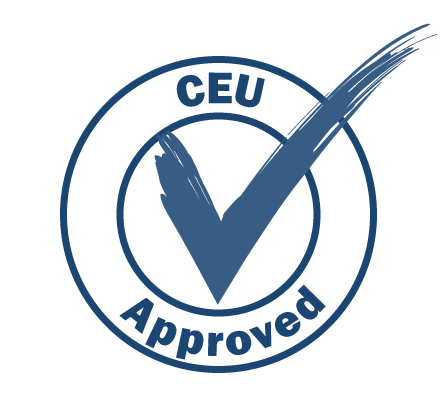
To make precision agriculture work, we need to gather a lot of critical information about the field. Unmanned aircraft systems (UAS) introduced an era of on-demand remote-sensing data collection at fine spatial and temporal resolutions. With the help of UAS, detailed maps of the field can be generated, which can help farmers see how their crops are doing and where they might be facing challenges. These maps can also show if the farming methods are working well or if they need to be adjusted. In this article, we’ve provided an overview of the current state-of-the-art UAS sensors and their applications, along with associated challenges and prospects of UAS mapping in agriculture. Earn 0.5 CEUs in Crop Management by reading this article and taking the quiz at https://web.sciencesocieties.org/Learning-Center/Courses.
Precision agriculture, often termed as site-specific crop management, is like farming with super intelligence. Instead of treating the whole crop field in the same way, each small part of the field is closely examined and managed according to its needs. It is important because different areas of the field can be very different from each other. Factors such as soil nutrient levels, soil physical and chemical properties, soil moisture level, and abiotic/biotic stress patterns can differ from one location to another. Adapting crop management practices and inputs based on the specific conditions of each location can help farmers save money, improve crops yields and quality, and protect the environment by using the right amount of nutrients and chemicals.
To make precision agriculture work, we need to gather a lot of critical information about the field. Remote sensing has emerged as an effective tool for collecting data at the field scale, but traditional approaches such as using remote sensors on towers come with limitations such as a fixed position from where data is collected. Furthermore, aircraft or satellite datasets also have spatial and temporal data resolution limitations. As such, unmanned aircraft systems (UAS) have proven to be an important tool to overcome these limitations of traditional remote sensing and introduce an era of on-demand remote sensing data collection at fine spatial and temporal resolutions. They're great because they can fly low and take clear pictures, and they can do it quickly.
With the help of UAS, detailed maps of the field can be generated, which can help farmers see how their crops are doing and where they might be facing challenges. These maps can also show if the farming methods are working well or if they need to be adjusted. For example, UAS with special cameras can identify issues with the plants that our eyes can't see, like diseases or stress. They can carry different sensors, like cameras that can see different colors or even thermal cameras that show heat patterns. This helps gather data on all kinds of crops and soil properties. When farmers have a better understanding of what's happening in the field, they can make smarter decisions. They can use just the right amount of water, nutrients, and pesticides as needed, which helps the crops grow better, saves money, and reduces harm to the environment.
As technology continues to improve, we'll see more and more UAS assisting in agriculture. They will become a common tool for farmers to make their fields healthier and more productive. In this article, we’ve provided an overview of the current state-of-the-art UAS sensors and their applications, along with associated challenges and prospects of UAS mapping in agriculture.
Type of Sensors
Unmanned aircraft systems can be equipped with different types of sensors that gather data on crops, soil, and the surrounding environment (Table 1). Following are the most common sensors used in UAS mapping:
Table 1. Commonly used UAS sensors in agricultural monitoring.
Sensor Type | Sensors | Bands | Number of |
---|---|---|---|
RGB | Parrot Sequoia, DJI Zenmuse XT2, Sony RX1R II | RGB | 3 |
Multispectral | MicaSense RedEdge, Parrot Sequoia, DJI Zenmuse Multispectral | RGB, red-edge, near-infrared | 4-12 |
Hyperspectral | Specim, Resonon, Headwall Photonics | RGB, near-infrared, shortwave-infrared | 100-200+ |
Thermal | FLIR Vue Pro, FLIR One X2, Seek Thermal Compact XR | Thermal | 1 |
aUsual number of bands in sensors.
RGB Sensors
RGB sensors are widely used in UAS mapping because they can capture images in the red, green, and blue color bands. These sensors are commonly used to create highly detailed maps of agricultural land. Since these three-color bands are visible to the human eye, the images they produce are easy for people to understand. Additionally, RGB sensors are relatively affordable and easy to use, making them a popular choice for farmers and crop consultants to monitor their fields. Even though RGB images may not provide as much detail as other options, several studies have shown that they are effective for estimating crop biomass and creating 3D models of field crops.
Multispectral Sensors
Multispectral sensors capture images in a wider range of bands (4 to 12 bands) than RGB sensors. This allows them to detect variations in plant health that are not visible to the naked eye and RGB sensors, such as chlorophyll content, water content, and nutrient levels, and provide more detailed information about crop health and stress levels. While multispectral sensors are more expensive than RGB sensors, they offer valuable insights into crop health. Multispectral cameras are categorized into two types: narrowband and broadband. Narrowband cameras have a smaller spectral range, which provides more precise spectral information. In contrast, broadband cameras, like the Sequoia with a 40-nm bandwidth, have wider spectral ranges that resemble those of satellites, allowing more convenient application of satellite-based sensor algorithms (e.g., vegetation indices) to broadband camera data.
Hyperspectral Sensors
Hyperspectral sensors capture images in hundreds or even thousands of bands spread across visible, near-infrared, and shortwave infrared bands, providing extremely detailed information about crop health and stress levels. However, adoption of hyperspectral sensors in precision agriculture is limited compared with other sensors due to multiple reasons. The vast amount of data generated by hyperspectral sensors, including hundreds of high- resolution spectral bands, requires specialized analysis and processing methods. The complexity of data acquisition and analysis also contributes to the scarcity of studies in this field as it demands specialized training. Additionally, hyperspectral sensors typically require larger UASs with higher payload capacities, limiting their application in large-scale fields. Furthermore, hyperspectral sensors are extremely expensive. Many remote-sensing experts anticipate that hyperspectral sensors will primarily serve research purposes. However, ongoing technological advancements are expected to lead to the development of smaller and more cost-effective devices, allowing hyperspectral-based sensing to become a mainstream approach in agriculture, forestry, and related fields.
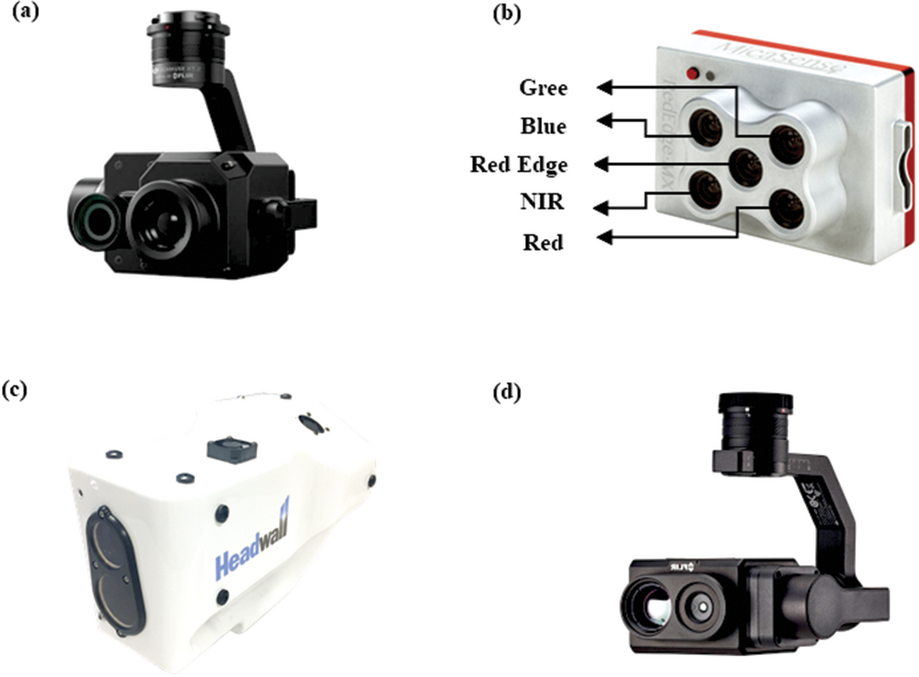
Thermal Sensors
Thermal sensors capture images of the thermal radiation/heat emitted by crops. Thermal UAS remote sensing in agriculture has been extensively studied, primarily focusing on detecting crop water stress and managing irrigation resources. Other applications include identifying plant pathologies, phenotyping, monitoring, yield estimation, and identifying drainage networks. However, the use of thermal sensors in UAS is not as extensive as other cameras due to limitations in geometric resolution and the nature of temperature data. Combining thermal cameras with RGB and multispectral sensors seems to enhance UAS capabilities in precision agriculture. Future developments in thermal remote sensing are needed to enhance user-friendliness and automation, simplifying data acquisition and processing.
Challenges
Despite the many potential benefits of utilizing UAS mapping for agriculture, its widespread adoption for agricultural sustainability faces a few challenges:
Practical Applications on Farmer Fields
One significant challenge facing the adoption of UAS in agriculture is their practical application on farms. While UAS have demonstrated their value in agricultural research and consultancy settings, their use by farmers remains limited due to several factors. Firstly, most farmers lack the technical knowledge and familiarity required to operate UAS effectively. The operation and maintenance of these devices can be complex, and the lack of expertise hinders their integration into everyday farming practices. Additionally, computational requirements for data processing and interpreting data are among major barriers. It is necessary to initiate educational programs, workshops, and training initiatives that can help bridge these knowledge gaps and empower farmers to effectively utilize UAS in their farming practices.
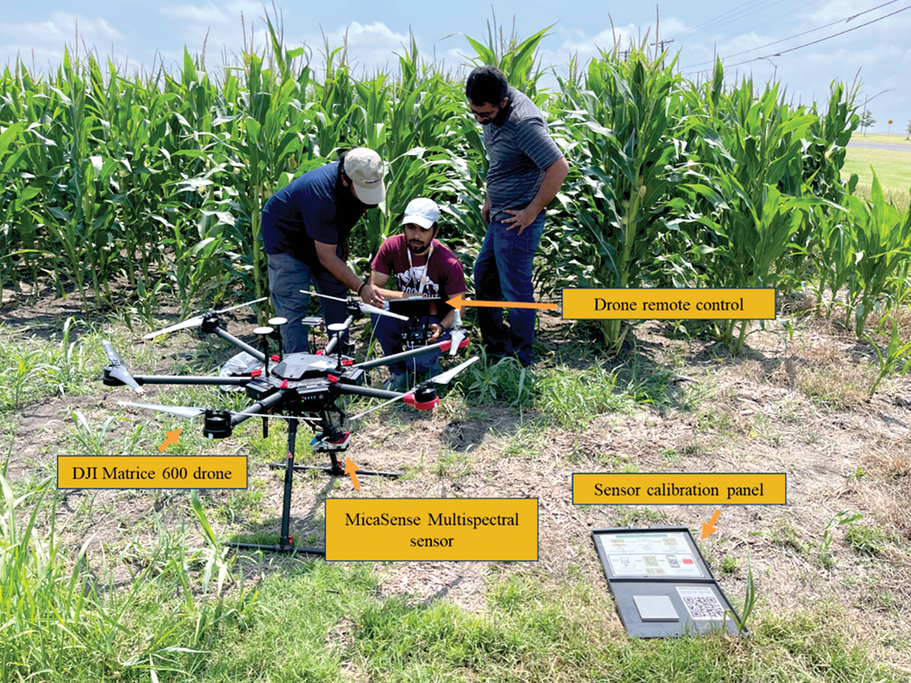
Secondly, the regulatory framework surrounding UAS mapping imposes certain restrictions and compliance requirements that need to be followed. Farmers must navigate through regulations related to flight altitudes, airspace restrictions, licensing, and privacy concerns. Compliance with these regulations can be challenging and may require additional time, effort, and resources. To address this challenge, it is important to simplify and tailor regulations specifically for the agricultural sector to facilitate compliance and encourage farmers to
embrace UAS technology.
The third significant factor affecting adoption by farmers is the cost associated with acquiring UAS and the required equipment. Unmanned aircraft systems and their accompanying sensors, software, accessories, and ongoing maintenance can be costly, making them inaccessible for many farmers, especially those with limited financial resources. Government agricultural and environmental agencies need to offer financial incentives or assistance programs to make UAS more affordable for farmers. This can help overcome the cost barriers associated with acquiring and maintaining these devices. Furthermore, collaborations among UAS manufacturers, agricultural organizations, educational institutes, and government agencies can play a crucial role in promoting the adoption of UAS in agriculture by addressing these limitations and creating a conducive environment for their successful implementation on farmer fields.
Reproducibility and Accuracy of UAS Mapping Data
Another critical challenge in the use of UAS for agriculture is ensuring the reproducibility and accuracy of the data collected through UAS mapping. Reproducibility involves capturing consistent and comparable data over time and across different areas. Several factors can affect data collection, leading to variations in outcomes and decision making. Weather conditions can have a significant impact on UAS data collection. Cloud cover, wind, and other environmental factors can affect the quality and consistency of data. How UAS are flown, including altitude and flight path, can influence data collection. Moreover, the quality of sensors used in UAS mapping plays a crucial role in data accuracy. Calibration and validation of sensors are essential to obtain reliable results.
Addressing these challenges requires a multidimensional approach. As a first step, standardization of data acquisition and processing methodologies can enhance reproducibility, allowing for consistent and comparable results such as collecting data during cloud-free days, near solar noon, and with a consistent flight altitude and overlapping plans. Rigorous sensor calibration, validation using ground truth measurements, and quality control measures can further enhance data accuracy and reproducibility.
Future Perspectives
Despite the challenges mentioned above, the outlook for UAS mapping in agricultural sustainability is promising with several potential advancements and applications on the horizon. Manufacturers need to focus on improving sensors for enhanced data acquisition, reducing costs, and developing user-friendly systems, making UAS mapping more accessible to farmers. Furthermore, the future scope of UAS mapping in agriculture lies in the integration of state-of-the-art techniques such as machine learning, artificial intelligence, and the internet of things (IoT). By leveraging these technologies, UAS mapping can be utilized for advanced applications in agricultural practices. Machine-learning algorithms can analyze large datasets collected by UAS to identify patterns and correlations, aiding in decision- making processes. Artificial intelligence can enable real-time data processing, facilitating on- demand monitoring and providing farmers with up-to-date information for timely interventions. Integrating UAS with IoT devices, such as weather sensors and crop health sensors, will create a comprehensive network of interconnected devices, enabling smart
farming practices.
Lastly, fostering the development of open access data platforms and promoting data sharingamong research institutions globally is essential. Currently, numerous research institutes are engaged in UAS-based agricultural research to varying degrees. However, these research endeavors tend to be exclusive, impeding the pace of digital advancement in agriculture compared with other industries. Therefore, it is crucial to prioritize data sharing and collaboration to establish standardized and robust methods for UAS operation, data processing, and analytics in precision agriculture applications.
Self-Study CEU Quiz
Earn 0.5 CEUs in Crop Management by taking the quiz for the article at https://web.sciencesocieties.org/Learning-Center/Courses. For your convenience, the quiz is printed below. The CEU can be purchased individually, or you can access as part of your Online Classroom Subscription.
- Studies of RGB sensors have shown that they are not effective for estimating crop biomass.
- True.
- False.
- Multispectral sensors can detect variations in
- heat emitted by crops.
- chlorophyll content.
- nutrient levels.
- B and C.
- All of the above.
- How many bands can hyperspectral sensors capture?
- Only 12 at a time.
- Only 50 at a time.
- 100–200+.
- 100 or less.
- Processing and interpretation of data from UAS requires a large amount of computational power, a barrier to widespread UAS adoption.
- True.
- False.
- Reproducibility, a critical challenge in the use of UAS for agriculture, means
- capturing consistent and comparable data over time and across different areas.
- offering financial assistance programs to make UAS more affordable.
- training farmers to interpret the data collected.
- educating farmers on how to navigate the regulations related to UAS.
Text © . The authors. CC BY-NC-ND 4.0. Except where otherwise noted, images are subject to copyright. Any reuse without express permission from the copyright owner is prohibited.